Projects & Software
Current research projects
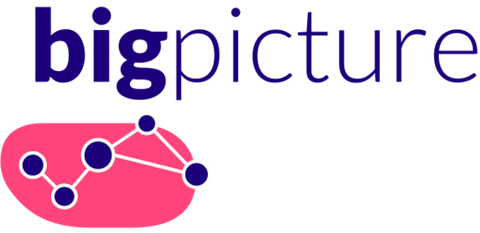
BIGPICTURE
The goal of Bigpicture is to accelerate the development of AI in pathology by providing a large repository of high-quality annotated pathology data, accessible in a responsible, inclusive and sustainable way.
Read more →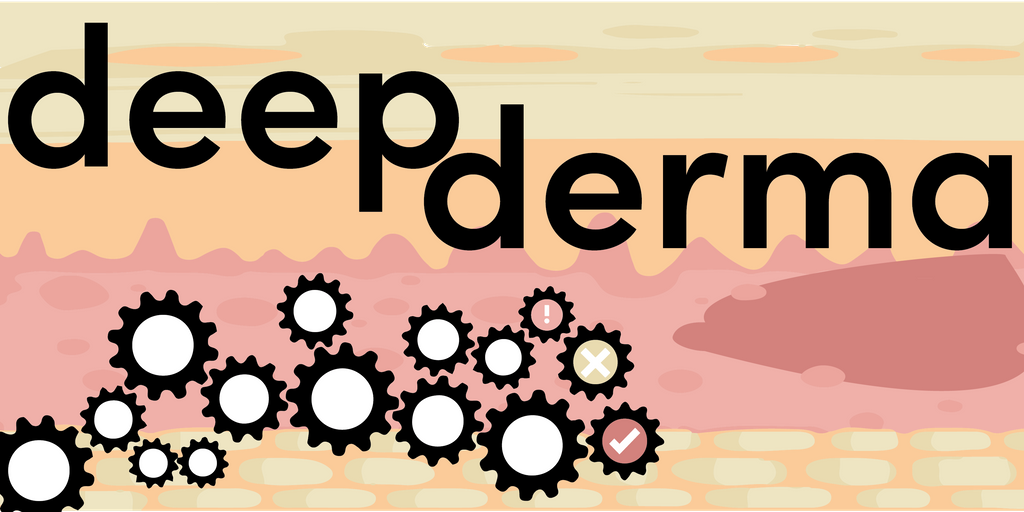
Deep Derma
The aim of this project is to apply artificial intelligence to detect basal cell carcinoma.
Read more →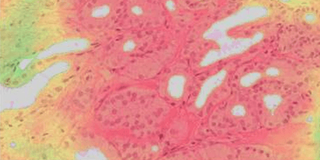
Deep PCA
In this project, we will combine deep learning and digitized whole-slide imaging of prostate cancer for reproducible extraction of quantitative biomarkers.
Read more →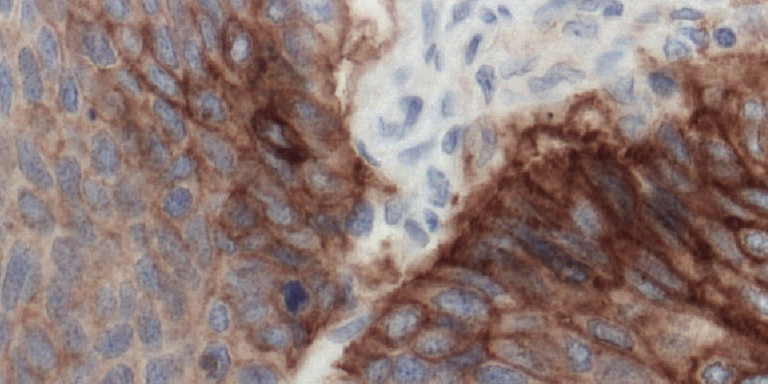
IGNITE
The goal of IGNITE is to use automatic biomarker extraction with deep learning to predict the response of non-small cell lung cancer patients to immunotherapy.
Read more →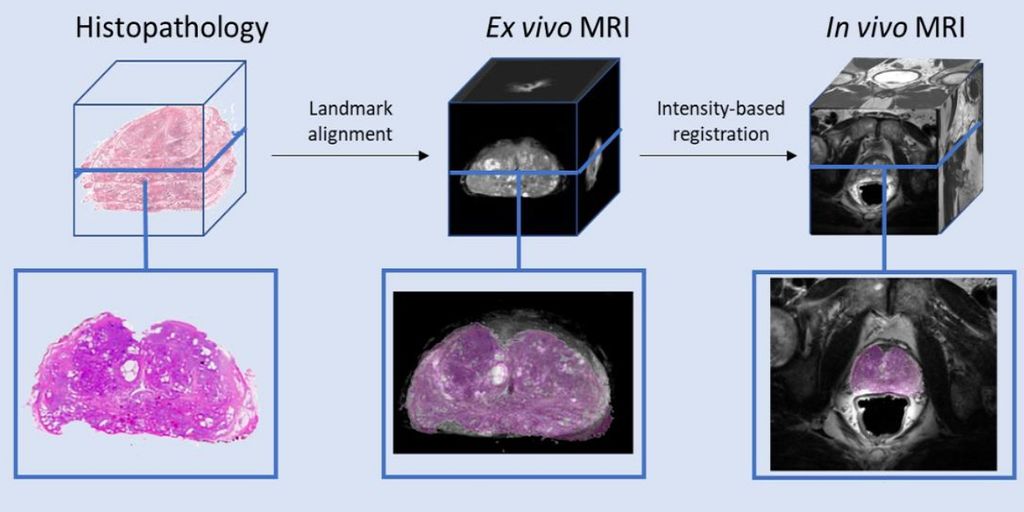
PathRad Fusion
The aim of this project is to integrate histopathological and radiological images to improve our understanding of disease diagnosis and progression in prostate cancer.
Read more →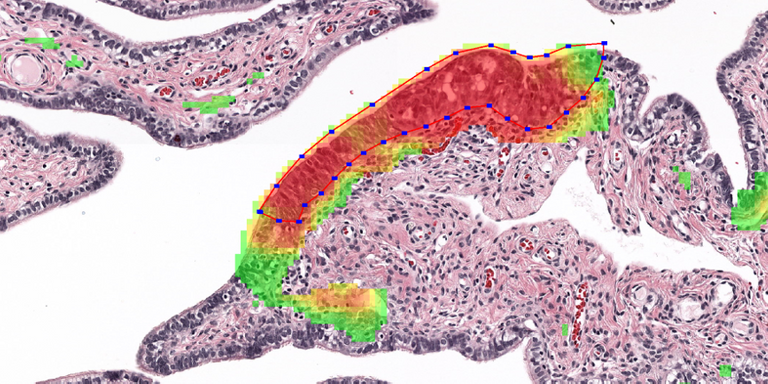
STIC
The aim of STIC is to improve the diagnostics of precursor lesions to high grade serous carcinoma (HGSC), the most common and lethal form of ovarian cancer.
Read more →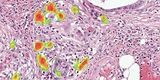
Tumor budding
In this project, we will develop and validate digital image analysis algorithms for quantification of tumor budding from scanned whole slide images.
Read more →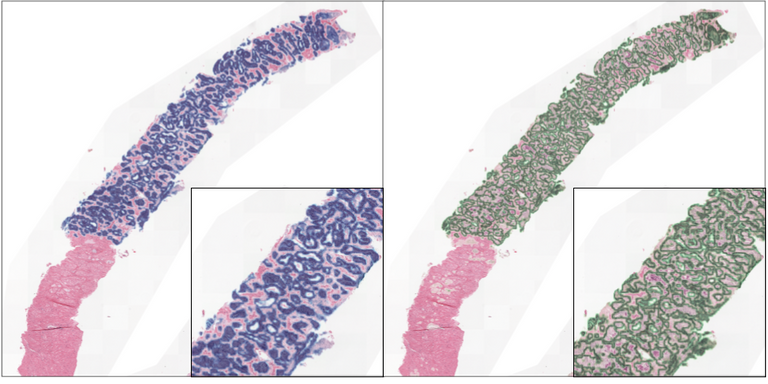
UNESCO
The aim of UNESCO is to study techniques for estimating uncertainty in computational pathology.
Read more →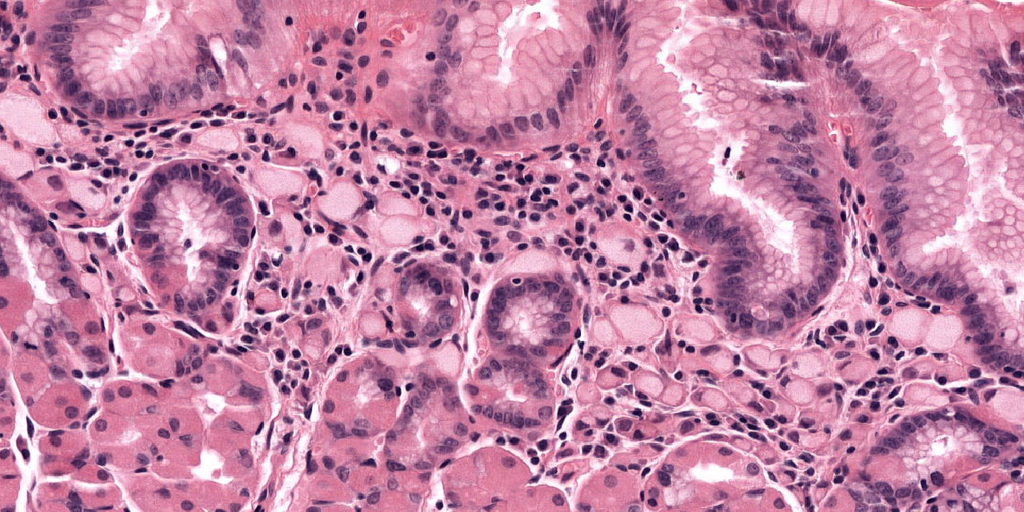
UNIC
The aim of UNIC is to develop artificial intelligence methods to refine diffuse-type gastric cancer (DGC) diagnostics.
Read more →Software
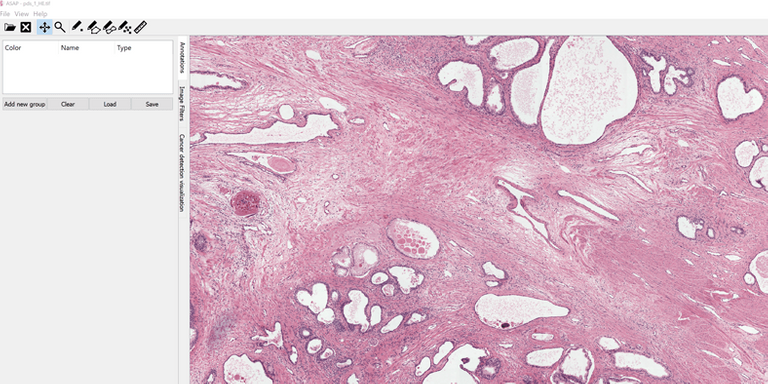
ASAP - Fluid whole-slide image viewer
ASAP (Automated Slide Analysis Platform) is a fast and fluid viewer for digitized multi-resolution histopathology images. ASAP offers several tools to make annotations in an intuitive way. Dots, rectangles, polygons are all supported. ASAP allows on-slide visualization of image analysis and machine learning results such as segmentation masks with customizable lookup-tables.
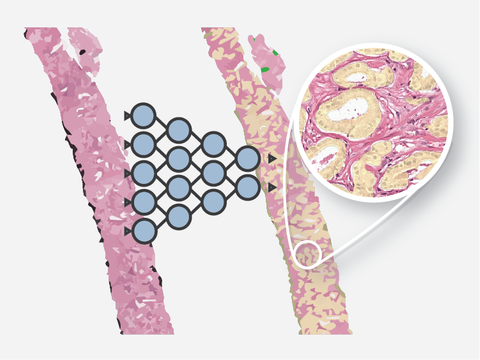
Automated Gleason Grading
Automated analysis of prostate biopsies following the Gleason grading system.
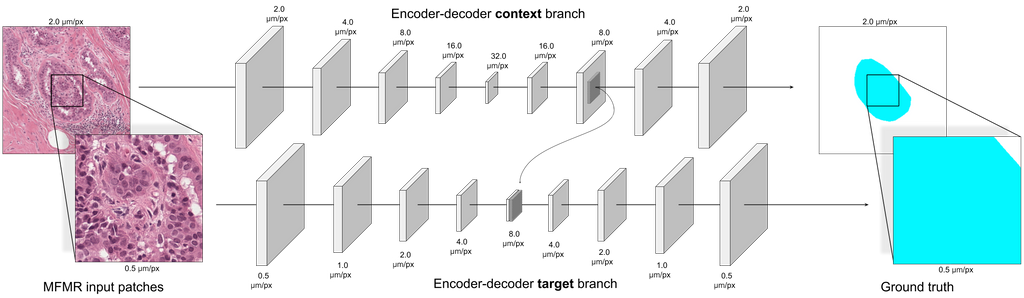
HookNet
HookNet: multi-resolution convolutional neural networks for semantic segmentation in histopathology whole-slide images
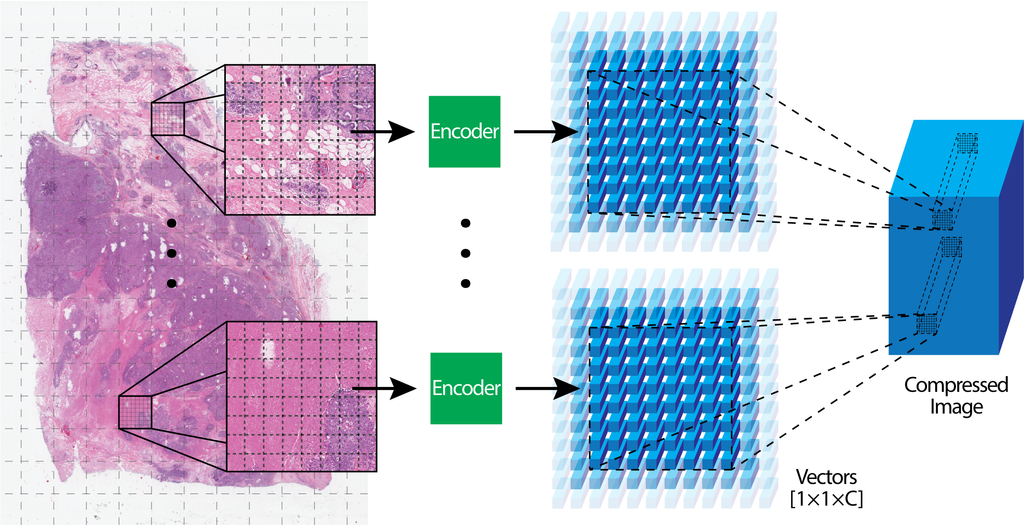
Neural Image Compression
Neural image compression for gigapixel histopathology image analysis.
Finished projects
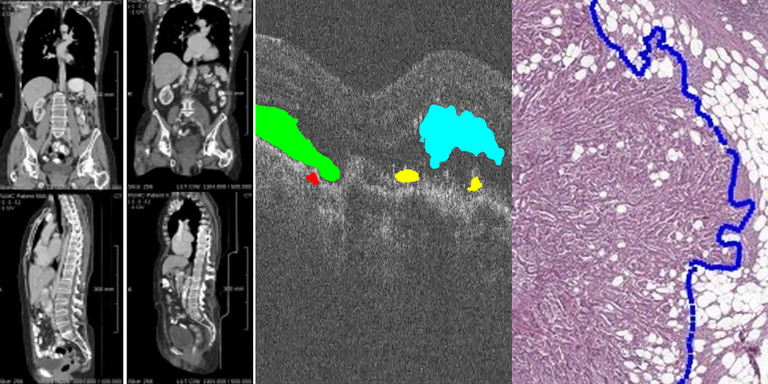
AMI
Automation in Medical Imaging - a joint project between DIAG and Fraunhofer MEVIS aimed at the development of a generic platform for automatic medical image analysis.
Read more →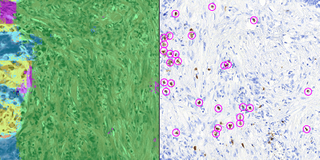
AQUILA
The goal of AQUILA is to investigate the prognostic value of Tumor Infiltrating Lymphocytes (TILs) in breast and colon cancer.
Read more →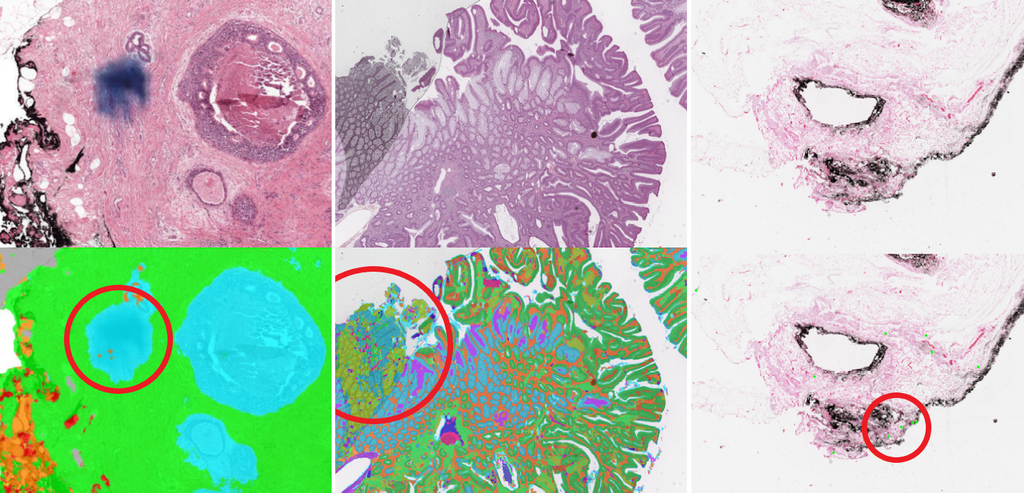
Artifact detection in digitized histopathology images
Development of a deep learning algorithm that can classify the different types of artifacts in whole slide images.
Read more →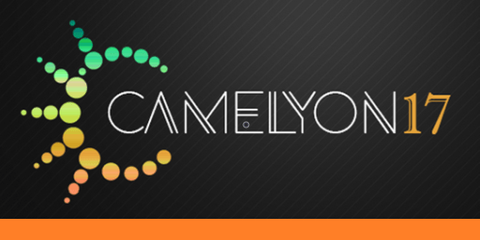
CAMELYON17
ISBI 2017 challenge to evaluate algorithms for automated detection and classification of breast cancer metastases in whole-slide images of histological lymph node sections.
Read more →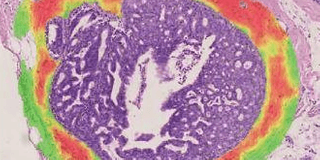
DCIS
The aim of this project is to use deep learning for histological assessment of the stroma for improved risk stratification of ductal carcinoma in situ (DCIS) patients.
Read more →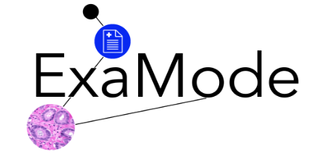
ExaMode
The aim of ExaMode is to collect training data with limited human interaction for the processing of exascale volumes of healthcare data.
Read more →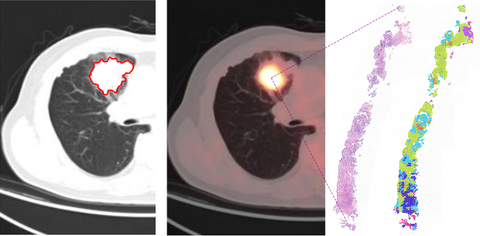
GENERATOR: Optimal treatment prediction for early stage lung cancer
We aim to combine advanced CT and FDG-PET image analysis with computational pathology to predict the most optimal treatment for each individual patient.
Read more →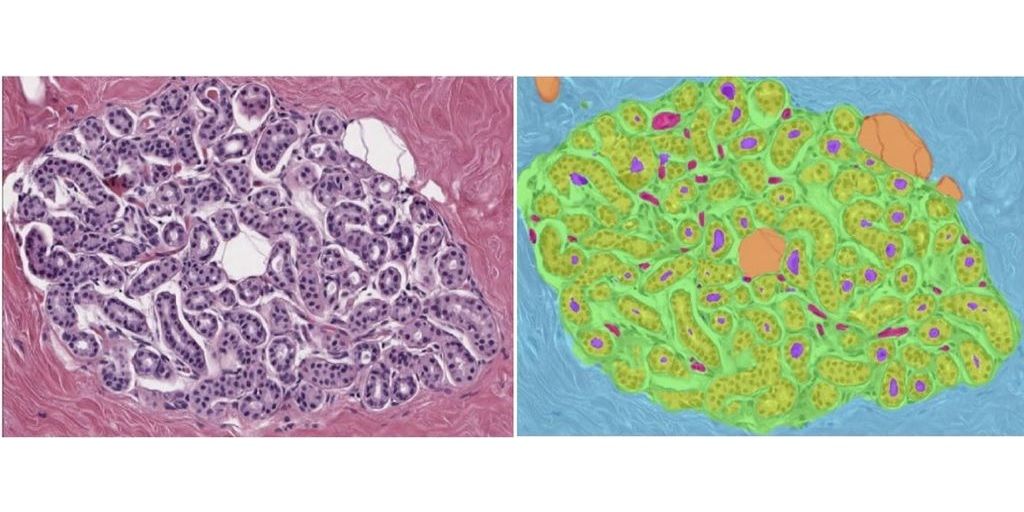
Identification of features in benign breast disease biopsies that predict breast cancer risk
Development of a deep learning system to predict BC risk in H&E
Read more →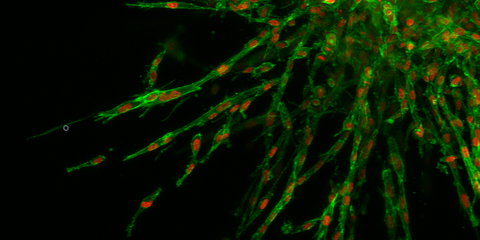
Multimot
MULTIMOT aims to build an open data ecosystem for cell migration research, through standardization, dissemination and meta-analysis efforts.
Read more →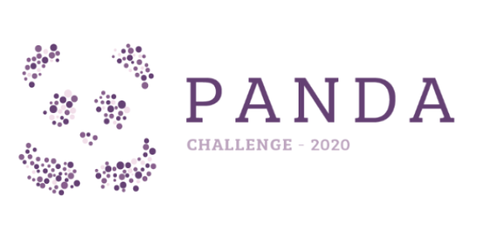
PANDA Challenge
Challenge on prostate cancer grading of biopsies using the Gleason grading system.
Read more →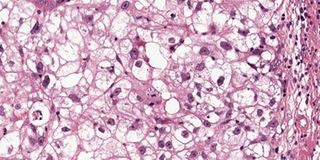
PRIM4BC
Detecting biomarkers for improved prognosis for triple negative breast cancer by combining histopathology, multiplex immunohistochemistry and Deep Learning.
Read more →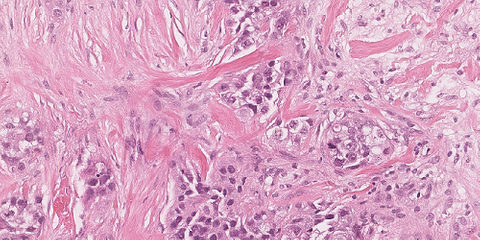
PROACTING
The aim of PROACTING is to predict neoadjuvant chemotherapy treatment response from a single pre-operative core-needle biopsy of breast cancer tissue.
Read more →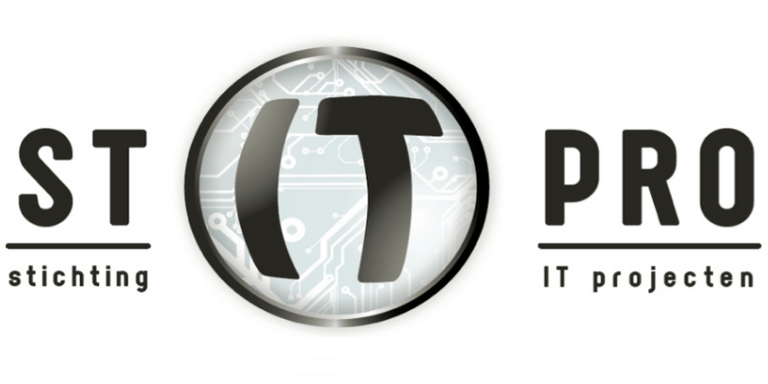
STITPRO I
Project on the development of ASAP and on the automatic detection of metastases in WSI of sentinel lymph nodes of breast cancer patients.
Read more →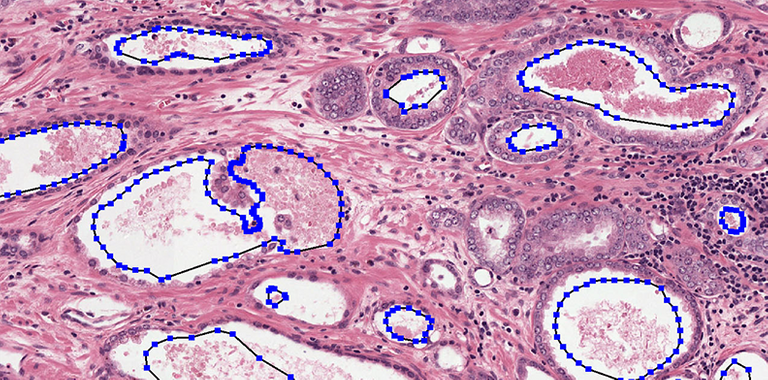
STITPRO II
Project with focus on the extension of open-source software ASAP that includes functionality for study and case management.
Read more →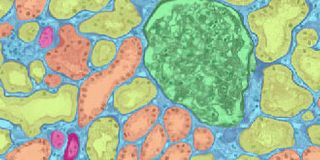
SysMIFTA
The investigation of the role of immune cell subsets in interstitial fibrosis and tubular atrophy in renal allografts, using multiplex immunohistochemistry and Deep Learning.
Read more →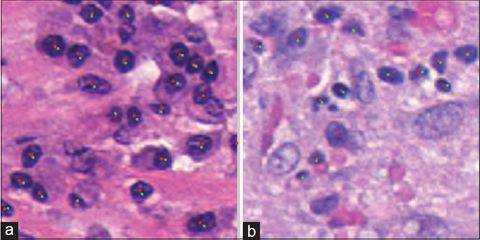
Automated Quantification of Tumor-Infiltrating Lymphocytes
Developing an algorithmn that can automatically detect and segment tumor-infiltrating lymphocytes in breast cancer.
Read more →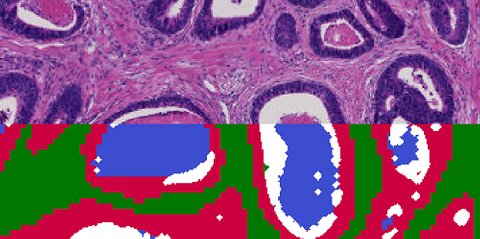
Tumor-Stroma Ratio
We aim at developing automatic and reproducible quantification of Tumor-Stroma Ratio in Whole-Slide Images using Deep Learning.
Read more →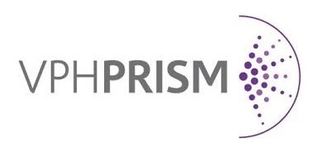
VPH-PRISM
Study on the correlations between several radiological modalities and histpathology for more understanding of breast cancer presentation.
Read more →